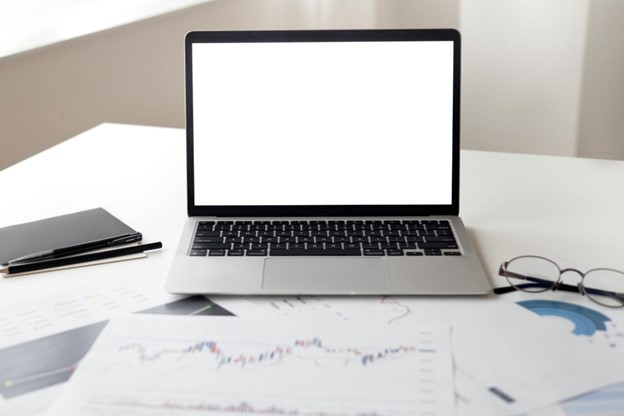
In an era defined by technological evolution, the financial sector stands at the forefront of embracing Artificial Intelligence (AI) to revolutionize its operations. As an integral component of the digital transformation journey, AI has swiftly permeated various facets of finance, offering solutions to longstanding challenges and paving the way for unprecedented opportunities. This article delves into the multifaceted landscape of AI in finance, exploring key applications, transformative technologies, real-world case studies, and the future trends that are set to reshape the industry.
Algorithmic Trading and High-Frequency Trading
Artificial Intelligence (AI) has revolutionized the financial landscape, particularly in the realm of algorithmic trading and high-frequency trading (HFT). These advanced technologies enable financial institutions to execute trades at unprecedented speeds, leveraging complex algorithms and machine learning models. The key advantage of AI in this domain lies in its ability to analyze vast amounts of market data in real-time, identify patterns, and execute trades with precision.
Algorithmic trading involves the use of algorithms to automate trading decisions, optimizing trade execution and minimizing human intervention. AI algorithms can analyze historical market data, identify trends, and make split-second decisions to buy or sell assets. This not only enhances the efficiency of trading strategies but also allows for the exploration of complex trading scenarios.
In the case of high-frequency trading, where speed is of the essence, AI algorithms can process market data at incredible speeds, enabling institutions to capitalize on fleeting opportunities. Machine learning models can adapt to changing market conditions and continuously refine trading strategies to stay competitive in dynamic financial markets.
As AI continues to evolve, the financial industry is witnessing a shift towards more sophisticated predictive models and neural networks. These technologies empower traders to make data-driven decisions, predict market movements, and optimize trading strategies for better returns.
Credit Scoring and Risk Management
Credit scoring and risk management are integral components of the financial industry, and AI has emerged as a game-changer in assessing creditworthiness and managing risks effectively. AI-powered credit scoring models leverage a diverse set of data, including traditional credit history, transactional data, and alternative data sources, to provide a more comprehensive and accurate evaluation of an individual’s or a business’s credit risk.
Machine learning algorithms can analyze large datasets and identify patterns that may not be apparent through traditional credit scoring methods. This allows financial institutions to extend credit to a broader range of individuals and businesses, fostering financial inclusion while maintaining a robust risk management framework.
In risk management, AI plays a crucial role in identifying and mitigating potential threats. Advanced analytics and machine learning algorithms can assess market conditions, detect anomalies, and predict potential risks in real-time. This proactive approach enables financial institutions to implement timely risk mitigation strategies, reducing the likelihood of financial losses.
AI-driven credit scoring and risk management not only streamline processes but also enhance the accuracy and efficiency of decision-making in the financial sector. As the volume and complexity of financial data continue to grow, AI’s adaptive capabilities position it as a cornerstone in shaping the future of credit assessment and risk management.
Machine Learning and Predictive Analytics
Machine Learning (ML) and predictive analytics have emerged as transformative technologies in the field of finance, reshaping the way financial institutions analyze data, make decisions, and manage risks. ML algorithms, fueled by vast amounts of historical and real-time data, enable the identification of complex patterns and trends that traditional analytics may overlook. In finance, this translates to more accurate predictions of market trends, customer behavior, and investment opportunities.
Predictive analytics, a subset of ML, leverages statistical algorithms and machine learning techniques to forecast future outcomes based on historical data. In finance, this technology is employed to predict market movements, assess credit risk, and optimize investment portfolios. Financial institutions utilize predictive analytics to make informed decisions, reduce uncertainty, and stay ahead in a highly dynamic and competitive market.
Machine learning models continuously learn and adapt to changing market conditions, enabling financial professionals to refine their strategies and respond swiftly to emerging trends. The ability to analyze vast datasets in real-time empowers financial institutions to make more accurate predictions, ultimately leading to better investment decisions and improved overall financial performance.
Natural Language Processing (NLP) for Sentiment Analysis
Natural Language Processing (NLP) is a transformative technology that focuses on the interaction between computers and human language. In finance, NLP is particularly valuable for sentiment analysis, a process that involves extracting insights and sentiments from textual data such as news articles, social media, and financial reports. By understanding the sentiment behind the text, financial institutions can gauge market sentiment, identify potential risks, and make more informed investment decisions.
NLP algorithms can analyze large volumes of unstructured data, extracting valuable insights about how market participants feel about specific assets, sectors, or economic conditions. This information is crucial for anticipating market trends, predicting price movements, and adjusting investment strategies accordingly. Sentiment analysis through NLP provides a valuable layer of intelligence that complements traditional financial analysis methods.
As the financial landscape continues to be influenced by information disseminated through various channels, NLP-powered sentiment analysis becomes increasingly vital. It empowers financial professionals to make timely and well-informed decisions, leveraging the wealth of information available in textual form across the digital landscape.
Robotic Process Automation (RPA) for Efficiency
Robotic Process Automation (RPA) is a transformative technology that has gained prominence in the financial industry for its ability to automate repetitive and rule-based tasks. In finance, RPA is deployed to streamline operational processes, enhance efficiency, and reduce manual errors. By automating routine tasks such as data entry, reconciliation, and report generation, RPA allows financial institutions to reallocate human resources to more strategic and value-added activities.
RPA in finance goes beyond simple task automation; it involves the use of software robots that can mimic human interactions with various systems and applications. These robots can handle complex workflows, ensure data accuracy, and execute tasks with precision, significantly reducing processing times. This not only improves operational efficiency but also contributes to cost savings and overall productivity.
The adoption of RPA in finance is driven by the need for increased efficiency and accuracy in a highly regulated and data-intensive industry. Financial institutions that integrate RPA into their processes can achieve operational excellence, enhance customer service, and gain a competitive edge in the rapidly evolving financial landscape.
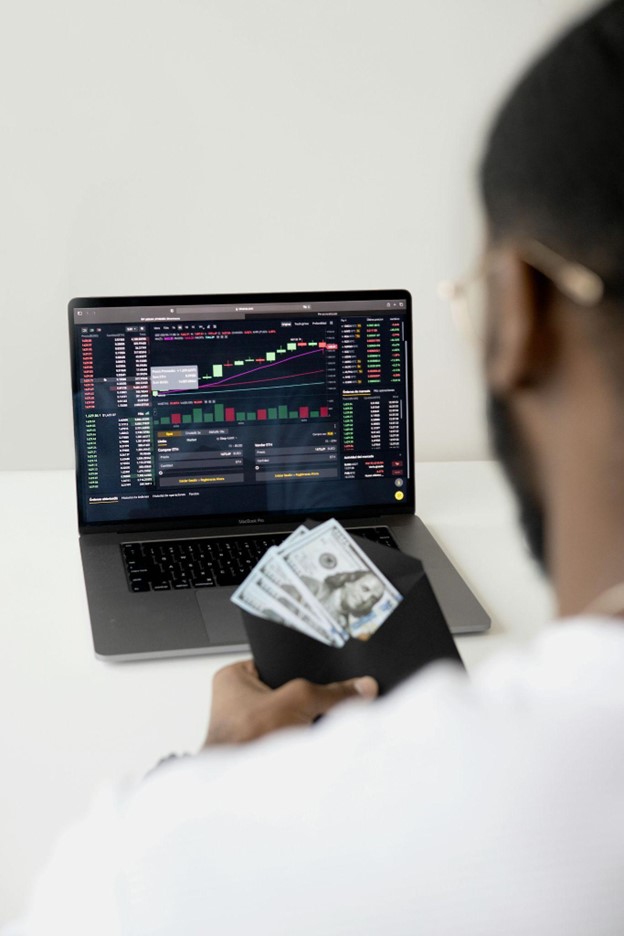
Blockchain and Smart Contracts for Security and Transparency
Blockchain technology and smart contracts have emerged as transformative forces in the financial sector, offering enhanced security, transparency, and efficiency in various processes. Blockchain, a decentralized and distributed ledger, ensures the immutability and security of financial transactions by recording them in a tamper-resistant manner. This technology eliminates the need for intermediaries, reducing the risk of fraud and enhancing the overall integrity of financial transactions.
Smart contracts, built on blockchain platforms, are self-executing contracts with predefined rules and conditions. In finance, smart contracts automate and enforce contractual agreements, reducing the need for manual intervention and minimizing the risk of errors or disputes. This not only accelerates transaction processes but also enhances trust among parties involved in financial transactions.
The decentralized nature of blockchain technology ensures that financial data is secure, transparent, and accessible only to authorized parties. This enhances data integrity and reduces the risk of cyber threats or unauthorized access. Blockchain’s impact on financial transparency is particularly evident in areas such as supply chain finance, cross-border payments, and securities trading.
As financial institutions seek innovative solutions to address security concerns and improve operational efficiency, the adoption of blockchain and smart contracts continues to gain momentum. These transformative technologies are poised to reshape the future of finance by providing a secure and transparent foundation for a wide range of financial transactions and processes.
Ethical Concerns and Bias in AI Algorithms
The integration of AI in finance brings forth ethical concerns and the potential for bias in algorithmic decision-making. As AI systems are trained on historical data, they may inadvertently perpetuate existing biases present in the data. For example, if historical lending data reflects biased lending practices, AI algorithms could perpetuate discrimination in credit scoring. Financial institutions must be vigilant in identifying and addressing these biases to ensure fair and ethical AI-driven decision-making.
Ethical concerns also arise in cases where AI algorithms are not transparent or understandable. The opacity of complex machine learning models can lead to a lack of accountability and trust. Financial institutions must prioritize transparency and explainability in their AI systems, providing stakeholders with insights into how decisions are made. Striking a balance between innovation and ethical considerations is paramount to building trust in the adoption of AI in finance.
Security and Data Privacy Implications
The adoption of AI in finance introduces security and data privacy implications that require careful consideration. As financial institutions increasingly rely on vast amounts of sensitive data, the risk of cyber threats and data breaches becomes a significant concern. AI systems, particularly those utilizing machine learning, are attractive targets for malicious actors seeking to manipulate models or gain unauthorized access to financial data.
Protecting customer data and ensuring compliance with data privacy regulations are critical challenges. Financial institutions must implement robust cybersecurity measures, encryption protocols, and access controls to safeguard AI systems and the data they process. Additionally, adherence to data protection regulations, such as GDPR, is imperative to avoid legal consequences and maintain customer trust.
Regulatory Hurdles and Compliance Issues
The financial industry operates within a highly regulated environment, and the adoption of AI introduces regulatory hurdles and compliance challenges. Regulators worldwide are grappling with the rapid advancements in AI and machine learning, seeking to establish frameworks that balance innovation with the need for consumer protection and financial stability. Financial institutions must navigate evolving regulatory landscapes, ensuring that their AI systems comply with existing and emerging regulations.
Compliance issues may arise due to the lack of standardized guidelines for AI applications in finance. Institutions deploying AI solutions must proactively engage with regulatory bodies, staying abreast of updates and working towards industry-wide standards. Implementing robust internal compliance measures is essential to mitigate the risk of regulatory scrutiny and legal consequences associated with non-compliance.
As the financial industry continues to harness the power of AI, addressing ethical concerns, ensuring data security, and navigating regulatory complexities are pivotal considerations. Financial institutions that prioritize these challenges will not only foster a responsible and ethical AI ecosystem but also position themselves as leaders in the evolving landscape of AI-powered finance.
FAQs
Q. What is the role of Artificial Intelligence in finance?
A. Artificial Intelligence (AI) plays a crucial role in finance by automating processes, enhancing decision-making, detecting patterns, and managing risk. It transforms traditional financial services and contributes to efficiency and innovation.
Q. How does AI improve data analysis in the financial sector?
A. AI enhances data analysis in finance by quickly processing vast amounts of data, identifying patterns, and extracting valuable insights. Machine learning algorithms help in predicting market trends, assessing risks, and making informed investment decisions.
Q. Can AI be used for fraud detection in the financial industry?
A. Yes, AI is highly effective in fraud detection. Machine learning models can analyze transaction patterns, detect anomalies, and identify potentially fraudulent activities in real-time, providing a proactive approach to combating financial fraud.
Q. What are robo-advisors, and how do they utilize AI in finance?
A. Robo-advisors are automated financial advisory platforms that use AI algorithms to provide investment advice and portfolio management. They leverage machine learning to analyze market trends, assess risk, and tailor investment strategies to individual client needs.
Q. How does Natural Language Processing (NLP) benefit financial institutions?
A. NLP allows financial institutions to process and understand human language. It facilitates sentiment analysis, helps in interpreting financial news and reports, and enables more accurate decision-making based on the information extracted from textual data.
Q. What is the impact of AI on algorithmic trading?
A. AI has a significant impact on algorithmic trading by automating the execution of trades based on predefined criteria. Machine learning models analyze market data in real-time, identifying patterns and making split-second decisions, leading to more efficient and adaptive trading strategies.
Q. How does AI contribute to personalized banking experiences?
A. AI enables personalized banking experiences by analyzing customer data to understand preferences, behavior, and financial goals. This information is used to offer tailored product recommendations, customized financial advice, and a more engaging overall banking experience.
Q. Are there any challenges associated with implementing AI in finance?
A. Yes, challenges include concerns about data privacy, regulatory compliance, ethical considerations, and the need for skilled professionals. Implementing AI in finance requires careful navigation of these challenges to ensure successful integration and operation.
Conclusion
In the grand tapestry of finance, Artificial Intelligence emerges as a transformative force, weaving together efficiency, accuracy, and innovation. From algorithmic trading to risk management, AI applications have redefined the contours of traditional financial practices, ushering in an era of unprecedented possibilities. However, with great potential comes great responsibility. As we navigate the intricate terrain of AI in finance, addressing ethical concerns, ensuring security, and embracing regulatory compliance become paramount. The future holds promise and challenges in equal measure, urging the industry to remain vigilant, collaborative, and forward-thinking. Through the continued synergy of human intellect and machine precision, the financial landscape is poised for a dynamic and AI-driven metamorphosis.